Research Library
As we believe in scientific research as the basis of our activities, we systematically mapped papers along different strategies and segments of private market investing.
Explore proprietary research of our co-founder Reiner Braun and that of the scientific community:
Transforming Private Equity Secondaries:
The private equity secondaries market has evolved from a niche segment into a crucial $100+ billion marketplace, representing approximately 10% of global private equity transactions. Yet, despite this growth, significant challenges remain in asset selection and pricing. This article demonstrates how artificial intelligence (AI) - particularly machine learning and natural language processing - is revolutionizing secondaries investing by uncovering predictive signals hidden in qualitative data that traditional metrics miss. Groundbreaking research examining thousands of GP reports across multiple funds reveals how the “tone” of management communications can predict future performance more accurately than conventional metrics alone. For investors navigating the complex secondaries market, embracing AI-driven approaches to augment human judgement is rapidly becoming not just an advantage but a necessity for sustainable competitive edge.
Limited Partners versus Unlimited Machines; Artificial Intelligence and the Performance of Private Equity Funds
We assemble a proprietary dataset of 395 private equity (PE) fund prospectuses to analyze fund performance and fundraising success. We analyze both quantitative and qualitative information contained in these documents using econometric methods and machine learning techniques. PE fund performance is unrelated to quantitative information, such as prior performance, and measures of document readability. Measures of fundraising success, in contrast, are correlated to most fund characteristics but are not related to future performance. Meanwhile, machine learning tools can use qualitative information to predict future fund performance: the performance spread between the funds within the top and bottom terciles of predicted probability of success is about 25%. Our findings support the view that in opaque and non-standardized markets, investors fail to incorporate qualitative information in their asset manager selection process, but do incorporate salient quantitative information.
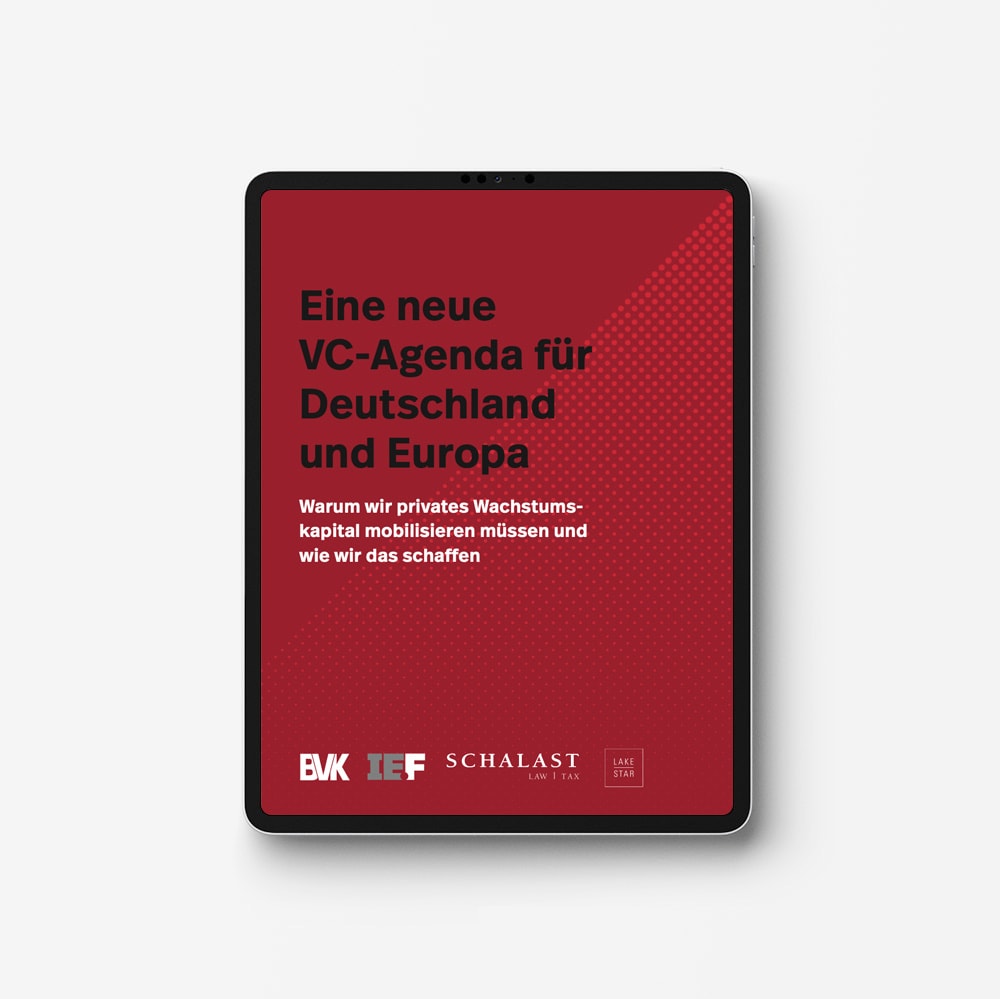
A new VC agenda for Germany and Europe
A new VC agenda for Germany and Europe - Why we need to mobilize private growth capital and how we can do it
In this study, we highlight the persistent major hurdles hindering access to venture capital, including a lack of private capital from institutional investors, internationally uncompetitive legal frameworks and a lack of opportunities for startup employees and citizens to participate in the value creation of startups and young companies.
Portfolio Construction in Venture Capital: The Impact of Portfolio Size on Risk and Returns
Returns in Venture Capital (VC) are characterized by extreme outliers and a notable number of defaulted transactions. Building a well-diversified portfolio is pivotal for investors to achieve sufficient downside protection without disproportionately trimming upside potential. This report simulates VC returns to understand the impact of portfolio size on risk and return potential. The simulations are based on the semicontinuous log-normal model of Juergens et al. (2022).
The analysis is conducted with 10,000 Monte Carlo simulations, each with a universe of 900 VC funds in total, which are equally distributed in early-stage (pre-seed and seed), mid-stage (Series A to Series B), and later-stage (Series C or later). That is 300 funds per stage focus and 18,000 transactions in total across all funds. The portfolio composition assumes equally-weighted portfolios of funds with 30 transactions in early-stage funds, 20 transactions in mid-stage funds, and 10 transactions in later-stage funds.
Do Entrepreneurs Make Good VCs
Using hand-collected data on the backgrounds of venture capitalists (VCs), we show that in a typical venture capital firm in our sample, 13.9% of VCs have been entrepreneurs before becoming a VC, referred to as entrepreneur VCs. Both OLS and 2SLS analyses suggest that venture capital firms employing a greater fraction of entrepreneur VCs have better performance. In addition, the positive effect of entrepreneur VCs on venture capital firm performance is stronger for smaller and younger venture capital firms, and venture capital firms specializing in high-tech industries and in early-stage investments. We further explore performance implications of VCs with prior experience in a finance-related field (i.e., Wall Street experience) and prior experience in a non-finance related field (i.e., Main Street experience). We find that contrary to prior experience in entrepreneurship, neither prior experience in Wall Street nor in Main Street is significantly related to venture capital firm performance. Finally, we provide evidence that entrepreneur VCs have greater individual performance in terms of VC rankings established by Forbes. Overall, our results are consistent with the idea that entrepreneur VCs have a better understanding of the business of starting and developing a new firm due to their first-hands experience, and play an important role in reducing the gaps in information and difference of opinions between an entrepreneur and the VCs backing the entrepreneur.
Do You Get What You See in Private Equity? A Bayesian Decomposition of Investment Skills
Analyzing a large sample of gross fund-level and deal-level returns in Private Equity (PE), we study systematic differences in investment skills across PE firms and what investors can learn about the true skill of PE firms from past performance. We extend the framework of Korteweg and Sorensen (2017) and establish a flexible variance decomposition model that estimates heterogeneity in returns, idiosyncratic risk-taking, and default risk. Our results show that investment skills are systematically different across PE firms with an estimated interquartile spread of returns ranging from 23% to 26% for deals and 17% to 21% for funds, relative to the market. Further, we find significant heterogeneity in idiosyncratic risk and default risk, but higher idiosyncratic variation does not explain higher expected returns. Since returns inherit substantial noise and spurious correlations from overlapping investments, investors require a considerable number of observations to learn about the true skill of PE firms.
Resilience and Cyclicality in Private Equity: Value Creation and Investment Flows in Economic Cycles
This study investigates the effects of economic cycles on abnormal value creation of buyouts (BO) and on the investment activity of the corresponding Private Equity (PE) funds. We benchmark a large sample of BO transactions with closely matched public companies from 1986 to 2017. Our results show that BO transactions have created significantly more value overall, but abnormal value creation has disappeared in more recent periods. However, BO transactions are considerably less sensitive to adverse shocks in the real economy than their public counterparts. The adverse impact of a 1% increased exposure to economic distress is between 0.4% and 0.5% lower for BO than for public benchmarks. Using the quarterly cash-flow data of the corresponding PE funds, we find that investment activity of initial fund flows is slightly pro-cyclical, while reinvestment activity is highly countercyclical to the real economy. Our results imply that PE funds act as liquidity providers during economic distress by providing 45% to 49% more capital to their existing portfolio companies than in undistressed periods.
University Venture Capital – The Promise and Pitfalls of University Direct Investments
Over the past three decades, universities in industrialized countries have become increasingly active as venture capital financiers. Here, we analyze if investments in university-affiliated portfolio companies, in the form of an institutional-personal relation between the university and the founders, are a commercially successful investment proposition. We use a hand-collected data set of 706 university portfolio companies in the United States and the United Kingdom to extend previous case-based evidence that investments in faculty- and student-led start-ups are an elusive promise that rarely pays off commercially. Furthermore, we provide evidence that geographic proximity to a top venture capital ecosystem is a highly performance-relevant characteristic for university investors.
International Evidence on Value Creation in Private Equity Transactions
Understanding value creation at the transaction level is at the heart of explaining private equity (PE) returns. Taking advantage of a proprietary sample of 2,029 international buyout deals executed between 1984 and 2013 we provide detailed evidence on financial, market and operational value creation drivers. Additionally, we unravel the differences in value creation between regions, industries, transaction sizes and over time, providing limited and general partners with the opportunity to compare their past transactions with those of their respective peer groups.
Financial Intermediation in Private Equity: How Well Do Funds of Funds Perform?
This paper focuses on funds of funds (FOFs) as a form of financial intermediation in private equity (both buyout and venture capital). After accounting for fees, FOFs provide returns equal to or above public market indices for both buyout and venture capital. While FOFs focusing on buyouts outperform public markets, they underperform direct fund investment strategies in buyout. In contrast, the average performance of FOFs in venture capital is on a par with results from direct venture fund investing. This suggests that FOFs in venture capital (but not in buyouts) are able to identify and access superior performing funds.
Follow the Money: How Venture Capital Facilitates Emigration of Firms and Entrepreneurs in Europe
An increasingly global venture capital (VC) business raises the question whether foreign VCs’ investments pull economic activity away from domestic economies. Using a large sample of VC-backed European ventures, we analyze whether involvement of foreign VCs influences firms’ and entrepreneurs’ migration patterns. We provide evidence that foreign investors, in particular from the U.S., on average, back much better European ventures and increase the likelihood of foreign exits and emigration of entrepreneurs. These effects are robust to endogenous selection. Our findings suggest that VC firms are a funnel through which high-impact economic actors are absorbed by countries with foreign VC presence. Governmental efforts to increase domestic supply of VC should have a positive impact on domestic economies.
Transferable Skills? Founders as Venture Capitalists
In this paper we explore whether or not the experience as a founder of a venture capital-backed startup influences the performance of founders who become venture capitalists (VCs). We find that nearly 7% of VCs were previously founders of a venture-backed startup. Having a successful exit and being male and white increase the probability that a founder transitions into a venture capital career. Successful founder-VCs have investment success rates that are 6.5 percentage points higher than professional VCs while unsuccessful founder-VCs have investment success rates that are 4 percentage points lower than professional VCs. While successful founder-VCs do get higher quality deal flow than professional or unsuccessful founder-VCs, observably higher deal quality does not explain the entire difference in performance. Using an instrumental variables approach to separate unobservable deal quality from value-add, we find that the outperformance of successful founder-VCs is consistent with them adding more value post-investment.
Benchmarking Venture Capital Databases
There has been an increasing asymmetry between the rising interest in private companies and the limited availability of data. While a group of new commercial data providers has identified this gap as a promising business opportunity, and has started to provide structured information on private companies and their investors, little is known about the quality of the data they provide. In this paper, we compare detailed and verified proprietary information on 339 actual venture capital (VC) financing rounds from 396 investors in 108 different (mostly European) companies, with data included in eight frequently used VC databases to help academic scholars and investors better understand the coverage and quality of these datasets and, thus, interpret the results more accurately. We find that greater financing rounds are more likely to be reported than lower ones. Similarly, financing round sizes and post-money valuations are more likely to be reported for greater financing rounds than for lower ones. Our analysis reveals that VentureSource, Pitchbook and Crunchbase have the best coverage, and are the most accurate databases across our key dimensions of general company data, founders and funding information. We describe our findings in detail and discuss potential implications for researchers and practitioners.
Venture Capital (Mis)Allocation in the Age of AI
We use machine learning to study how venture capitalists (VCs) make investment decisions. Using a large administrative data set on French entrepreneurs that contains VC-backed as well as non-VC-backed firms, we use algorithmic predictions of new ventures’ performance to identify the most promising ventures. We find that VCs invest in some firms that perform predictably poorly and pass on others that perform predictably well. Consistent with models of stereotypical thinking, we show that VCs select entrepreneurs whose characteristics are representative of the most successful entrepreneurs ( i.e., characteristics that occur more frequently among the best performing entrepreneurs relative to the other ones). Although VCs rely on accurate stereotypes, they make prediction errors as they exaggerate some representative features of success in their selection of entrepreneurs (e.g., male, highly educated, Paris-based, and high-tech entrepreneurs). Overall, algorithmic decision aids show promise to broaden the scope of VCs’ investments and founder diversity.
Adverse Selection and the Performance of Private Equity Co-Investments
Investors increasingly look for private equity managers to provide opportunities for co-investing outside the fund structure, thereby saving fees and carried interest payments. In this paper we use a large sample of buyout and venture capital co-investments to test how such deals compare with the remaining fund investments. In contrast to Fang, Ivashina and Lerner (2015) we find no evidence of adverse selection. Gross return distributions of co-investments and other deals are similar. Co-investments generally have lower costs to investors. We simulate net returns to investors and demonstrate how reasonably sized portfolios of co-investments significantly out-perform fund returns.
Human Versus Computer: Benchmarking Venture Capitalists and Machine Learning Algorithms for Investment Screening
I conduct an investment screening performance benchmarking between 111 venture capital (VC) investment professionals and a supervised gradient boosted tree (or “XGBoost”) classification algorithm to create trust in machine learning (ML) -based screening approaches, accelerate the adoption thereof and ultimately enable the traditional VC model to scale. Using a comprehensive dataset of 77,279 European early-stage companies, I train a variety of ML algorithms to predict the success/failure outcome in a 3- to 5-year simulation window. XGBoost algorithms show particularly excellent performance in terms of accuracy and recall, which denote the most important metrics in my setup. I benchmark the performance of the selected algorithm against that of the VC investment professionals by providing equal information in the form of 10 company one-pagers via an online survey and requesting respondents to select the five most promising companies for further evaluation. In addition to finding characteristic- specific performance dependencies for VCs, I find that the XGBoost algorithm outperforms the median VC by 25% and the average VC by 29%. Although I do not suggest replacing humans with ML-based approaches, I recommend an augmented solution where intelligent algorithms narrow down the upper part of the deal-flow funnel, allowing VC investment professionals to focus their manual efforts on the lower part of the funnel. Using this approach, they can rely on a scalable but objective pre-selection and focus their manual resources on evaluating the most promising opportunities and putting themselves into the best position to secure these deals.
The persistent effect of initial success: Evidence from venture capital
We use investment-level data to study performance persistence in venture capital (VC). Consistent with prior studies, we find that each additional initial public offering (IPO) among a VC firm’s first ten investments predicts as much as an 8% higher IPO rate on its subsequent investments, though this effect erodes with time. In exploring its sources, we document several additional facts: successful outcomes stem in large part from investing in the right places at the right times; VC firms do not persist in their ability to choose the right places and times to invest; but early success does lead to investing in later rounds and in larger syndicates. This pattern of results seems most consistent with the idea that initial success improves access to deal flow. That preferential access raises the quality of subsequent investments, perpetuating performance differences in initial investments.
Skill and Luck in Private Equity Performance
We use investment-level data to study performance persistence in venture capital (VC). Consistent with prior studies, we find that each additional initial public offering (IPO) among a VC firm’s first ten investments predicts as much as an 8% higher IPO rate on its subsequent investments, though this effect erodes with time. In exploring its sources, we document several additional facts: successful outcomes stem in large part from investing in the right places at the right times; VC firms do not persist in their ability to choose the right places and times to invest; but early success does lead to investing in later rounds and in larger syndicates. This pattern of results seems most consistent with the idea that initial success improves access to deal flow. That preferential access raises the quality of subsequent investments, perpetuating performance differences in initial investments.
Investor Personality and Success in VC
Our study introduces venture capital (VC) investors’ personality as a new perspective on investment performance. We assemble a sample of 911 VC investors with 8,258 investments and apply novel text analysis methods to assess investors’ personality traits based on their Tweets. Drawing on interactionist perspectives of the personality– performance relationship, we develop and empirically test theory on the impact of VC investors’ personality traits on investment success. We find that extraversion relates positively, while agreeableness and conscientiousness relate negatively, to the likelihood of a successful exit. By disentangling treatment and selection effects, we find that personality is primarily related to investors’ ability to create more value for the venture post-investment rather than select higher quality ventures ex-ante."
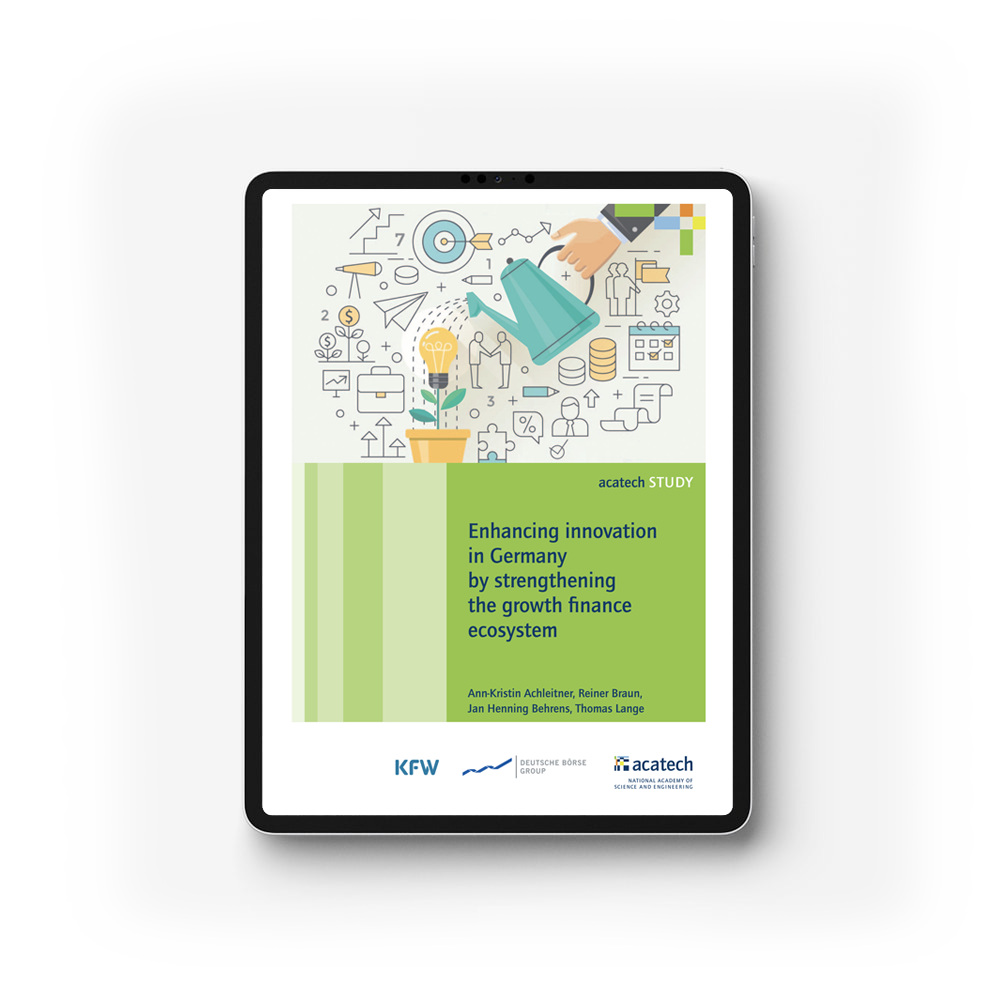
Enhancing Germany's Innovation Power – Bolstering the Ecosystem for Growth Financing
Lack of capital for high-tech growth companies is a major weakness in Germany’s innovation system, becoming a critical competitive disadvantage especially during digital transformation. This transformation demands radical technological innovations, new business models, and rapid growth. Acatech, in collaboration with KfW and Deutsche Börse, brought together various stakeholders from the financial sector, high-tech growth companies, industry leaders, and academics to assess the situation and develop actionable strategies for policy, business, and academia.